
What is the R1-Omni?
R1-Omni is developed by Alibaba Tongyi Labs, amultimodalLarge Language Model, which applies verifiable reward reinforcement learning (RLVR) methods to a fully modal large language model (LLM) for the first time. The model focuses onemotion recognitiontasks that can integrate multimodal information such as video, audio, and text for more accurate and interpretable sentiment analysis.The launch of R1-Omni marks an important advancement in the field of multimodal learning and interpretable AI.
R1-Omni Main Features
- Multimodal emotion recognition: R1-Omni is capable of processing multimodal data including video, audio and text, and realizes accurate emotion recognition through deep and reinforcement learning techniques.
- explanation of the reasoning process: The model not only outputs sentiment recognition results, but also provides a detailed inference process that explains how the model integrates information from different modalities to arrive at a prediction, enhancing the interpretability of the model.
- Strong generalization ability: R1-Omni also performs well on out-of-distribution datasets, with strong generalization capabilities to tackle unseen scenarios and tasks.
R1-Omni Core Technology
-
Verifiable Rewarded Reinforcement Learning (RLVR)::
- RLVR is a new training paradigm that centers on the idea of directly evaluating model outputs using a validation function without relying on a separate reward model in traditional reinforcement learning with human feedback (RLHF).
- In R1-Omni, RLVR is used to optimize model parameters to improve the accuracy and generalization of sentiment recognition.
-
Multimodal data processing::
- The R1-Omni is capable of handling multiple types of data such as video, audio and text, and through multimodal fusion technology, it enables the full integration and utilization of information.
- The model employs advanced feature extraction and coding methods to convert data from different modalities into a unified representation, which facilitates subsequent sentiment analysis and inference.
-
Reasoning Process Interpretation Techniques::
- R1-Omni explains how the model integrates information from different modalities to arrive at predictions by generating outputs that contain the inference process.
- This technique enhances the interpretability of the model, enabling users to better understand the decision-making process of the model and increasing the trust and usability of the model.
R1-Omni Usage Scenarios
- marketing: R1-Omni can analyze users' emotional tendencies towards video ads and provide advertisers with precise marketing strategies.
- Social Media Analytics: The model monitors user sentiment on social media, helping organizations understand public opinion and brand image.
- film and television production: R1-Omni is able to analyze viewers' emotional responses to video content such as movies and TV shows and provide producers with suggestions for improvement.
- mental health: In the field of mental health, models can assist doctors with sentiment analysis and help patients better understand and manage their emotions.
R1-Omni Performance Review
The researchers conducted a comprehensive performance review of R1-Omni, comparing it to several baseline models. The experimental results show that R1-Omni outperforms the comparison models in three aspects: reasoning ability, comprehension ability and generalization ability. The specific performances are as follows:
- Enhanced reasoning skills: R1-Omni provides a more coherent, accurate, and interpretable reasoning process with significantly improved reasoning power compared to the original baseline model.
- Improved comprehension: On the emotion recognition task, R1-Omni outperforms the comparison model in terms of accuracy and recall, showing better understanding.
- Greater ability to generalize: On the out-of-distribution dataset, R1-Omni also shows excellent generalization ability, with both WAR and UAR boosting over 131 TP4T.
R1-Omni Project Address
paper address::https://arxiv.org/abs/2503.05379
GitHub Address::https://github.com/HumanMLLM/R1-Omni
Model Download Address::https://www.modelscope.cn/models/iic/R1-Omni-0.5B
data statistics
Relevant Navigation

The third generation of artificial intelligence models developed by Musk's xAI company, with superior computational and reasoning capabilities, can be applied to a variety of fields such as 3D model generation and game production, which is an important innovation in the field of AI.

Ovis2
Alibaba's open source multimodal large language model with powerful visual understanding, OCR, video processing and reasoning capabilities, supporting multiple scale versions.

DeepSeek-V3
Hangzhou Depth Seeker has launched an efficient open source language model with 67.1 billion parameters, using a hybrid expert architecture that excels at handling math, coding and multilingual tasks.

XiHu LM
Westlake HeartStar's self-developed universal big model, which integrates multimodal capabilities and possesses high IQ and EQ, has been widely used in many fields.
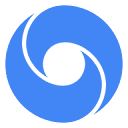
WebLI-100B
Google DeepMind launches a 100 billion visual language dataset designed to enhance the cultural diversity and multilingualism of AI models.

ZhiPu AI BM
The series of large models jointly developed by Tsinghua University and Smart Spectrum AI have powerful multimodal understanding and generation capabilities, and are widely used in natural language processing, code generation and other scenarios.

360Brain
360 company independently developed a comprehensive large model, integrated with multimodal technology, with powerful generation creation, logical reasoning and other capabilities, to provide enterprises with a full range of AI services.

SenseNova
Shangtang Technology has launched a comprehensive big model system with powerful natural language processing, text-born diagrams and other multimodal capabilities, aiming to provide efficient AI solutions for enterprises.
No comments...